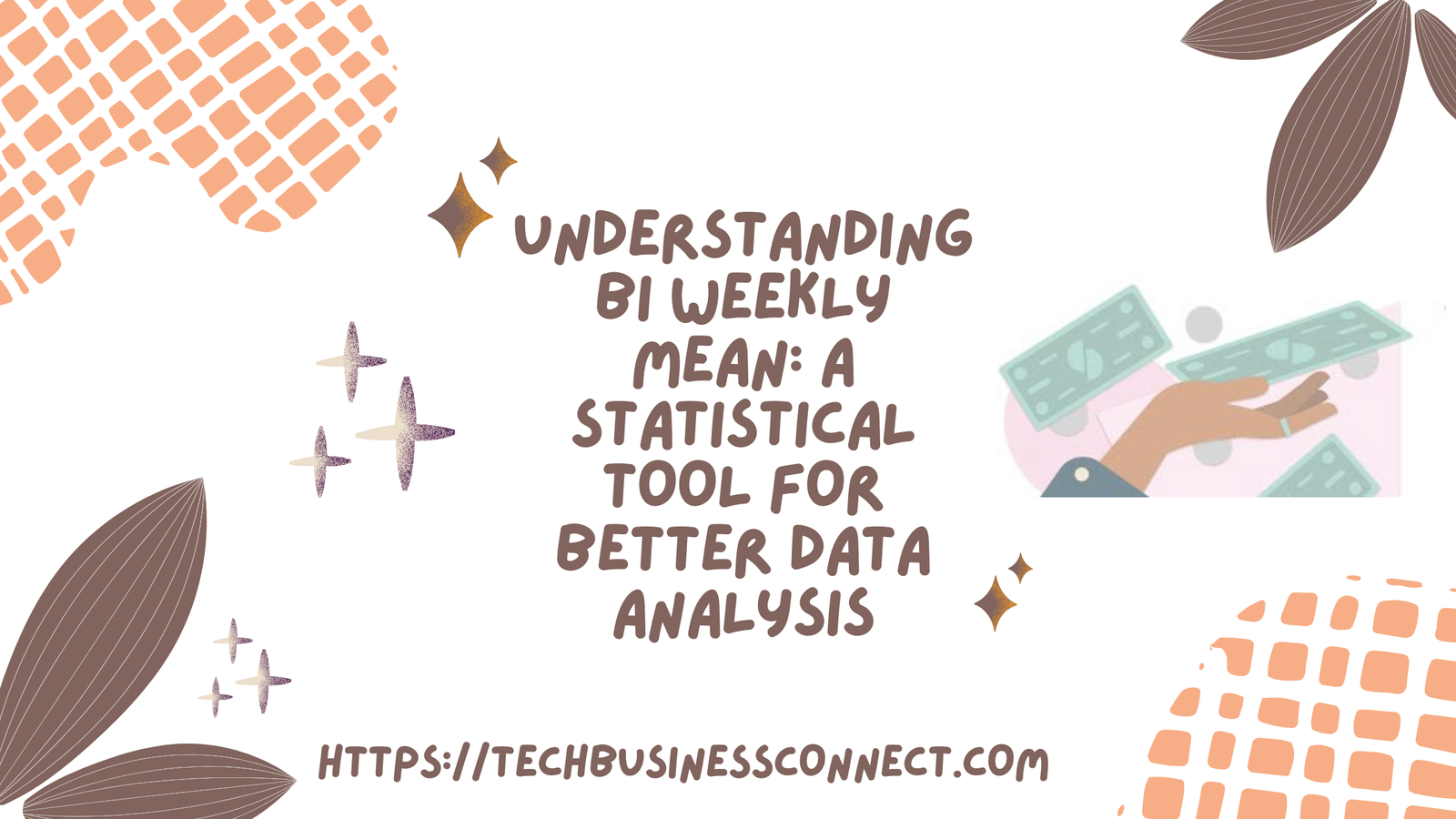
Understanding Bi Weekly Mean: A Statistical Tool for Better Data Analysis 2024
The concept of a “bi weekly mean” is foundational in statistics, often serving as a basic tool to understand the average behavior of data. A specific application of this concept is the bi weekly mean, which involves calculating the average of data collected over two-week intervals. This approach is particularly useful in contexts where data varies on a weekly basis but needs smoothing or averaging over a slightly extended period for insights. Let’s dive into the meaning of a bi weekly mean, its importance, how to calculate it, and its applications in various fields.
What is Bi Weekly Mean?
A bi weekly mean is the average value of a dataset collected over a two-week period. It is particularly valuable for summarizing data that fluctuates weekly but still follows a broader trend. For example, if you collect daily temperature data, revenue, or any other metric for a business, calculating the mean over a two-week span provides a middle ground between daily noise and monthly averages.
The concept is useful in any domain where data collected over shorter intervals requires stabilization or smoothing, helping researchers and analysts identify broader trends without losing the temporal relevance of weekly data.
Importance of Bi-Weekly Mean
The bi weekly mean is a statistical tool that has various practical advantages:
- Reducing Daily and Weekly Fluctuations: Many datasets contain daily or weekly variations, often affected by external factors like weather, social events, or seasonal fluctuations. Averaging data over two weeks allows us to see beyond the “noise” of these frequent changes, making it easier to discern underlying trends.
- Improving Decision-Making: For businesses or organizations that rely on consistent analysis of metrics like sales, productivity, or inventory, bi-weekly means can highlight changes that weekly or daily reports might miss. This can be helpful in resource allocation, stock planning, or even workforce management.
- Providing a Middle Ground for Analysis: Monthly data may be too broad, hiding shorter-term patterns, while weekly data may exaggerate short-lived fluctuations. A bi weekly mean serves as a compromise, revealing trends while retaining enough temporal resolution to remain responsive.
- Highlighting Medium-Term Trends: For researchers or analysts studying seasonal or periodic data, a bi-weekly mean helps bridge the gap between short-term noise and long-term trends. For example, temperature variations, economic data, or health statistics may show more accurate trends on a bi-weekly scale than on a daily or monthly scale.
How to Calculate the Bi Weekly Mean
Calculating a bi-weekly mean is a straightforward process, but it requires careful attention to ensure data is properly segmented and averaged over consistent two-week intervals.
- Collect Data: Start with a dataset that is segmented by a smaller unit of time, such as daily or weekly figures. Ensure that data collection is accurate and consistent, as errors in the raw data will skew the results.
- Organize Data by Two-Week Periods: Divide the dataset into two-week intervals. If your data is collected daily, for instance, aggregate it so that each two-week period forms a single group.
- Calculate the Mean for Each Interval: For each two-week period, sum up the data points and then divide by the number of data points in that period to get the average. If you have 14 daily data points, sum them up and divide by 14 to get the bi-weekly mean.
- Record and Analyze the Results: Once you have calculated the bi weekly means for each interval, you can plot these values or analyze them to understand trends over time. Graphing these values can provide a clear visual representation of changes over time and reveal trends or seasonal variations.
Examples of Calculating Bi Weekly Mean
Let’s consider a few practical examples where bi weekly means are calculated for different applications.
Example 1: Bi Weekly Temperature Mean
Suppose you have collected daily temperature readings over the course of two months. Organizing these readings into two-week periods allows you to calculate a bi-weekly mean that reflects the average temperature over each interval.
- Segment Data: Separate the daily readings into four two-week periods.
- Calculate Mean for Each Period: For each two-week period, sum the temperatures and divide by the number of days (14).
- Interpret the Results: The bi weekly means can show temperature trends without the daily fluctuations, helping meteorologists identify patterns in seasonal changes or anomalies.
Example 2: Bi-Weekly Revenue Mean for a Business
A retail business collects daily revenue data to understand its financial performance. To observe trends without the impact of daily sales volatility, the company can use a bi weekly mean.
- Segment Data: Separate the daily revenue figures into two-week intervals.
- Calculate Mean for Each Interval: For each two-week period, sum up the daily revenues and divide by 14.
- Analyze Results: By plotting these bi weekly means, the business can understand sales trends, assess the effectiveness of marketing efforts, and make data-driven decisions about stock and workforce management.
Applications of Bi-Weekly Mean in Various Fields
Bi weekly means are useful in multiple fields where data analysis benefits from medium-term averaging. Here’s a look at some prominent applications:
1. Healthcare and Epidemiology
In public health, tracking trends in data such as infection rates, mortality rates, and other health indicators can be critical. For instance, during flu season, calculating bi weekly means for new cases or hospitalizations can reveal trends in disease spread, helping public health officials allocate resources effectively.
2. Climate Science
Meteorologists and climate scientists often analyze data on a bi weekly scale to track temperature trends, precipitation levels, or other weather-related variables. This method smooths out daily or weekly anomalies that might otherwise skew data, providing a more accurate view of short-term climate patterns.
3. Business and Finance
In finance, bi weekly means can be useful for tracking stock prices, interest rates, or even consumer spending. Shorter intervals like weekly averages can be too volatile, while monthly averages might fail to capture important patterns. The bi-weekly mean provides a compromise, offering insight into stock performance or spending trends without short-term “noise.”
4. Agriculture
For farmers and agricultural researchers, tracking crop growth metrics, soil moisture levels, or pest populations on a bi weekly basis provides a good balance between real-time data and seasonal trends. This bi-weekly data can be used to make predictions and guide farming decisions, from irrigation scheduling to pest control.
5. Education and Workforce Management
Schools, universities, and companies often track metrics such as attendance, productivity, or student performance on a bi-weekly basis. A bi weekly mean smooths out individual absences or low-performance days, offering a clearer picture of overall trends in attendance or performance, which can then be used for interventions or policy adjustments.
Benefits and Limitations of Bi-Weekly Mean
While bi-weekly means offer numerous benefits, there are also some limitations to consider:
Benefits
- Smoothed Data: Reduces the noise of daily or weekly fluctuations, revealing underlying patterns.
- Enhanced Decision-Making: Useful in fields where medium-term trends are more informative than daily changes.
- Better Temporal Resolution than Monthly Data: Monthly averages may miss important changes, while bi-weekly means balance data smoothing with temporal relevance.
Limitations
- Loss of Granular Data: Averaging data over two weeks means that specific variations within each period are lost, which could be significant in some cases.
- Potential Bias: If data trends or patterns change abruptly within a two-week period, the bi-weekly mean may mask important details.
- Dependency on Accurate Data Collection: Errors in daily or weekly data points are aggregated and can impact the bi-weekly mean, so data quality must be high.
Bi-Weekly Mean vs. Other Averages
To put bi-weekly mean in context, it’s useful to compare it with other types of averages:
- Daily Mean: Provides the most granular information but is highly susceptible to fluctuations. It’s helpful for real-time analysis but can be misleading if there’s high volatility.
- Weekly Mean: Smooths out daily fluctuations but may still be sensitive to events or anomalies within a week.
- Monthly Mean: Provides a longer-term trend and reduces noise, but may be too broad for tracking medium-term changes.
The bi-weekly mean is a middle ground between the weekly and monthly mean, offering insights that neither extreme can provide.
Conclusion
The bi-weekly mean is a valuable statistical tool, providing insights that daily, weekly, or monthly averages alone might miss. Whether in healthcare, business, finance, or environmental science, calculating and interpreting bi-weekly means allows analysts and researchers to better understand medium-term trends, providing actionable insights and facilitating improved decision-making.